AI Bias- an Issue impeding AI in Governance
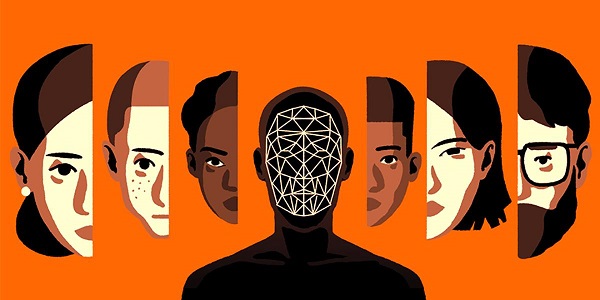
In June, India’s MeitY released the Draft National Data Governance Framework Policy to improve access, quality and the use of non-personal data in line with the emerging technology needs. It is a key step towards a wider adoption of AI technology, in various areas, including governance. However, AI bias is a concern the needs addressing before India takes the plunge.
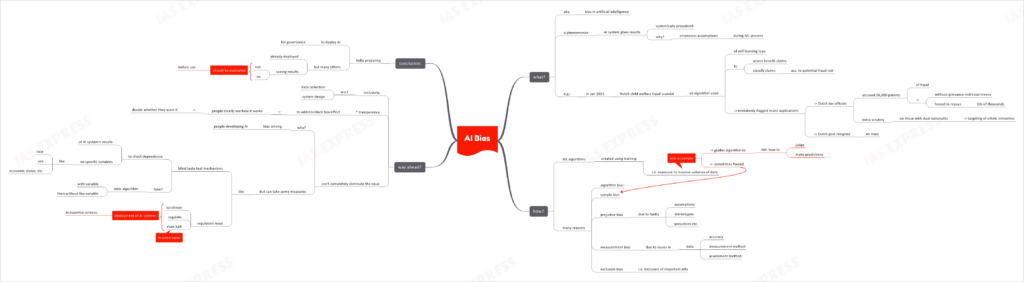
This topic of “AI Bias- an Issue impeding AI in Governance” is important from the perspective of the UPSC IAS Examination, which falls under General Studies Portion.
What is AI bias?
- AI bias or bias in artificial intelligence is a phenomenon in which the AI system gives results that are systemically prejudiced. This occurs because of erroneous assumptions made during the machine learning process.
An Example:
- In January 2021, the Dutch government had to resign en mass following a child welfare fraud scandal.
- An algorithm was deployed to detect misuse of the benefits. This ‘self-learning’ algorithm assessed benefit claims and classified them based on the potential fraud risk.
- However, it mistakenly flagged many applications, resulting in the tax authorities accusing some 26,000 parents of fraudulently availing child allowance over the years.
- The parents were left without any means of redress and were forced to repay massive amounts. Many with dual nationality were subject to extra scrutiny, resulting in disproportionate targeting of ethnic minorities.
How does it occur?
- Machine learning algorithms is an important part of the technology. These are created using the concept of ‘training’. During this training process, the system is exposed to a large collection of data which is used as a sample to determine how it can judge/ make predictions.
- AI biases occur due to anomalies in the algorithm’s output. This may be caused due to various reasons:
- Algorithm bias- due to problems in the algorithm
- Sample bias- due to problems in the data used for training the machine learning model
- Prejudice bias- due to faulty assumptions, stereotypes, prejudices, etc. in the data
- Measurement bias- due to problems with the accuracy, measurement/ assessment method of the data
- Exclusion bias- due to exclusion of important data points
How can it be addressed?
- Inclusivity is a key part of the solution. Inclusivity is required in the context of system design as well as data collection.
- Improved transparency would help address the ‘black box effect’ in AI technology. When people are able to clearly see how these systems work, they can make informed decisions on whether it would suit their needs.
- While it is impossible to completely weed out AI bias, given the inherent prejudices among the people developing it, some measures can be taken. For instance, the blind taste test mechanism:
- It is a mechanism to check whether the results from an AI system are dependent on specific variables like race, sex, economic status, etc.
- The mechanism does this by first testing the algorithm with the variable and then without it.
- In the meantime, regulators must scrutinize and regulate (or even halt in some cases) the deployment of AI systems in essential services.
Conclusion:
Even as India prepares to deploy AI technology for governance purposes, other countries have already deployed and seeing results- both positive and negative- from these systems. It would be in our best interest to evaluate these systems for biases before using them in essential service deliveries.
Practice Question for Mains:
What is AI bias? Why does it occur? How can it be addressed? (250 words)